AI and brainwaves: Using AI to decode messages in our brain
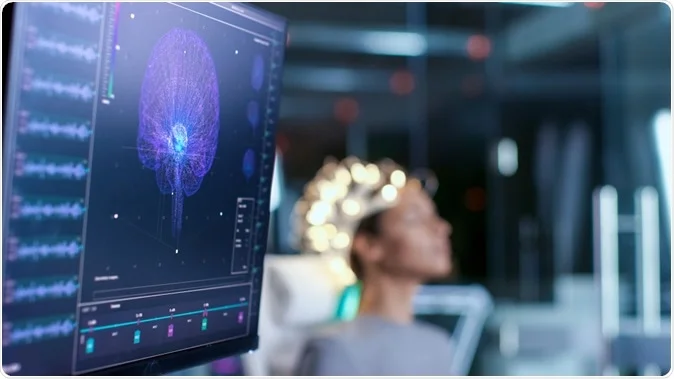
Introduction
In the realm of understanding brain activity, we've already developed sophisticated tools like EEG (Electroencephalography) and MEG (Magnetoencephalography) to capture and interpret brainwaves. These machines detect electrical or magnetic signals generated by the brain's neurons firing. EEG records electrical activity via electrodes placed on the scalp, while MEG measures magnetic fields produced by neural activity. In short, these signals come from the brain's cells talking to each other. By understanding these signals, we learn how the brain works and what happens when we think, feel, or move. It's like tuning into the brain's own radio station to decode its messages. Every thought, feeling and movement a human has or conducts produces very specific EEG and MEG data, meaning that these values can differ based on very small changes in stimuli (images, touch or speech).
Imagine you are sitting in a chair with a screen in front of you. The screen flickers and you are shown an image of an apple. When you look at the apple, specific regions of your brain that process shape, color, and recognition of objects get activated. This activation leads to certain groups of neurons firing in a particular way, creating unique patterns in the EEG and MEG data. The screen flickers again, but now you are shown an image of an cat instead. When you switch to viewing the cat, different brain areas related to visual recognition of animals, shapes, or emotions might get activated, resulting in a different set of patterns in the EEG and MEG data.
Looking at this example, we can start to imagine that EEG and MEG data is certainly ideal from a deep learning perspective. The data is numeric, it is categorizable (the brain state of looking at a cat compared to an apple) and produces large amounts of data through its various sensors (high-dimensional data). In this post, we will be focusing on two breakthroughs in speech and image decoding where researchers put this to the test.
Speech decoding using recordings
Scientists at the University of Texas have managed to create and train a model (integrated with ChatGPT-1) based on research subjects listening to about 16 hours of recorded stories of various magnitudes. This whilst an fMRI-scanner (Functional Magnetic Resonance Imaging) was registering their brain acitivity in real-time. By doing this, they were trying to map a representation of brain activity to the sentence or word spoken in the recoding. Using this data, the main research question was:
Can AI produce and predict what the subject listened to by just using data from the subjects brain activity?
The results were highly successful, where the researchers found that the model not only captured the meaning of the recording but some times even the exact phrase that the subject was exposed to. This means that if a subject was listening to the sentence "The sun gently peeked above the horizon", the model not only had the capability (in some cases) to produce the exact sentence but also that the meaning of the sentence was that it represented a sunrise.
The model was by no means perfect but had a remarkable ability to decode central motives of the recordings, as shown below:
The research also showed great results when decoding from video to text. This meant that using just the brain activity of the subject watching a video, the model could accurately create a text representation of what was going on in the video itself.
Real life applications
The implications of this research could be significant for the future, especially for people who have lost or have difficulties with verbal communication. It opens up the possibility for people who are mentally conscious but unable to communicate to use their brainwaves as a bridge when interacting with other people. However frightening the idea of an AI accurately being able to put your thoughts into text (hoping for an on/off button here), it is a fascinating discovery that advances our understanding of the brain.
Image recreation using image decoding
In October of 2023, researchers at Meta pushed this field of research even further. By using just MEG data, they managed to create a model that could now decode visual representations in the brain. The research showed that it could accurately recreate an image from the subjects brain activity:
To the left is a picture that a subject was shown and to the right is the image that the AI predicted that the subject was looking at. The AI had no idea what the person was looking at, but used only the subjects brainwaves to predict the image.
Summary
As an AI optimist, these two examples of groundbreaking research not only push AI-research further but also make significant contributions to neuroscience and the understanding of the human brain. In a total sum game, the research is not only fascinating but is a positive contribution to both fields.
However, AI moving into the decoding of brain waves can be a frightening scenario and sounds eerily similar to a wide range of sci-fi plots on the more dystopian side of things. Therefore unsurprisingly, 2023 also marked the starting point for the extensive work with regulating the AI-industry with main ambassadors such as Sam Altman of OpenAI encouraging and welcoming the regulation.
As mentioned earlier, 2023 was truly the year of AI. It was also the start of my own journey experimenting with deep learning models, creating simple AI-projects using pytorch, Kaggle and Pandas. Just by working with these models on a basic level, it is abundantly clear that the range and the depth of possibilities regarding AI is endless. That is precisly why we in parallel should push for supervision and tight regulation of the field as a whole.
Thank you for reading and happy holidays!
Sources
Toward a real-time decoding of images from brain activity - Meta
Paper: Semantic reconstruction of continuous language from non-invasive brain recordings